Computer-Aided Drug Design Methods PMC
Table Of Content
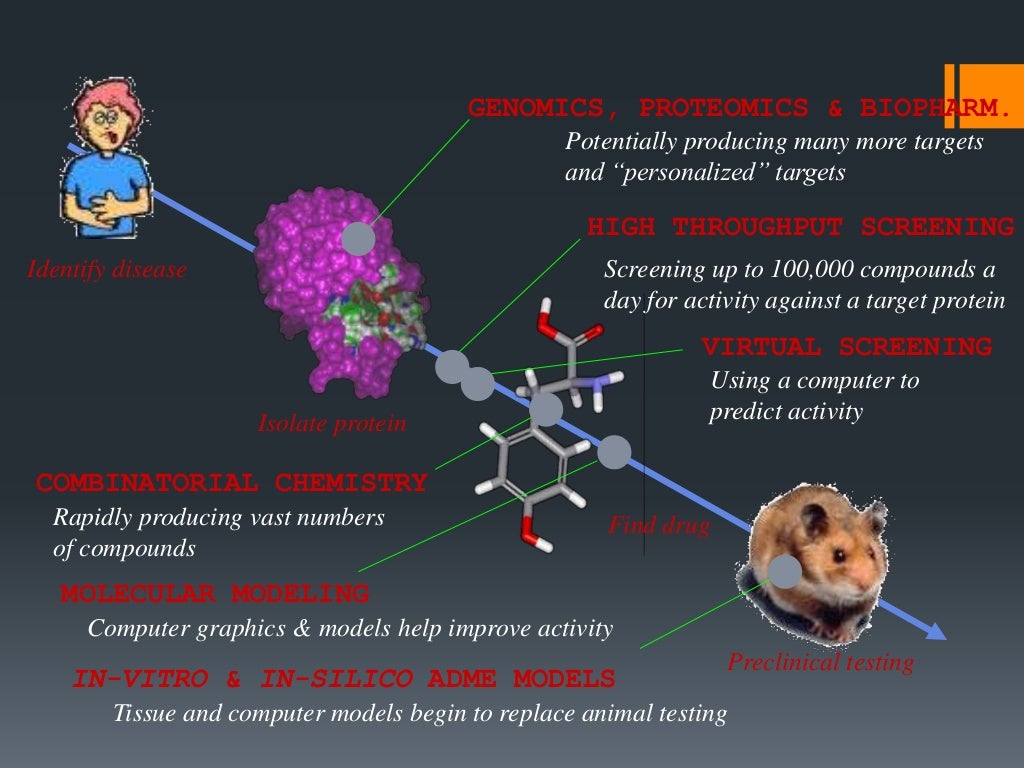
The amount of known/unknown data set used for developing the molecular structure along with its activity towards any target. Computer-aided drug design (CADD) approaches are playing an increasingly important role in understanding the fundamentals of ligand-receptor interactions and helping medicinal chemists design therapeutics. About 5 years ago, we presented a chapter devoted to an overview of CADD methods and covered typical CADD protocols including structure-based drug design (SBDD) and ligand-based drug design (LBDD) approaches that were frequently used in the antibiotic drug design process. Advances in computational hardware and algorithms and emerging CADD methods are enhancing the accuracy and ability of CADD in drug design and development. In this chapter, an update to our previous chapter is provided with a focus on new CADD approaches from our laboratory and other peers that can be employed to facilitate the development of antibiotic therapeutics. Data-driven approaches have a long history in drug discovery, in which ML algorithms such as support vector machine, random forest and neural networks have been used extensively to predict ligand properties and on-targets activities, albeit with mixed results.
Principal Scientist - CADD
For SBDD, protein 3D structure is required to explore atomic level details of the ligand-protein interactions. When no protein structure is available from the PDB, structure prediction methods such as homology modelling (92) were used traditionally to generate 3D models. With the surging of AI and related DL techniques, DL driven structure prediction methods such as RoseTTA fold (48) and AlphaFold (49) can now predict most protein 3D structures to a level of approaching experimental accuracy. In the recent challenging 14th Critical Assessment of protein Structure Prediction (CASP14), AlphaFold was demonstrated to greatly outperform other methods, and its predictions are competitive with experimental structures in a majority of cases.
Outlook towards computer-driven drug discovery

The results obtained showed inhibitors had low cytotoxicities, suggesting potential for drug development [82]. Inhibition of these two enzymes constitutes treatment for neurological diseases like autism spectral disorder, Alzheimer, and fragile X syndrome. Alokam et al. reported the successful use of CADD to design dual inhibitors for these enzymes [76], by employing a combination of pharmacophores and using a molecular docking approach to identify chemical entities. In vitro validation of selected chemical entities demonstrated their inhibitory potentials against ROCK-I and NOX2. Structural information about an identified target is a prerequisite for SBDD, but the structures of several identified neurodegenerative drug targets have yet not been determined [32, 33].
How a computer can help in drug design and discovery?
Chemical spaces of gigascale and terrascale, provided that they maintain high drug likeness and diversity, are expected to harbour millions of potential hits and thousands of potential lead series for any target. Moreover, their highly tractable robust synthesis simplifies any downstream medicinal chemistry efforts towards final drug candidates. Determining the structure of a target molecule follows the identification of a specific drug target [29]. Despite the availability of advanced techniques, the structures of a large number of proteins have not been identified [30]. Homology modelling helps in this situation because it can be used to generate the structures of proteins on information available for similar proteins [31]. An updated view on different types of NDs, their effect on human population and a brief introduction to CADD, various approaches involved in this technique, ranging from structural-based to ligand-based drug design has been discussed.
B. Ligand-based drug designing (LBDD)
The application of rational drug design as an integral part of CADD provides useful insights into the understanding of the binding affinity and molecular interaction between target protein and ligand. Additionally, lead identification in pharmaceutical research has been facilitated by the availability of supercomputing facility, parallel processing, and advanced programs, algorithms, and tools [7]. Furthermore, recent advancements in artificial intelligence (AI) and machine learning methods have greatly aided in analyzing, learning, and explaining the pharmaceutical-related big data in the drug discovery process [8].
Lead optimization and its strategies
This problem persists despite the availability of large number of antibiotic drugs, indicating the need for more novel antibiotic drug classes to overcome the resistance problem (1, 2). Computer-Aided drug design (CADD) approaches are playing an increasingly important roles in understanding the fundamentals of ligand-receptor interactions and helping medicinal chemists design therapeutics. About five years ago, we presented a chapter devoted to an overview of CADD methods and covered typical CADD protocols including structure-based drug design (SBDD) and ligand-based drug design (LBDD) approaches that were frequently used in the antibiotic drug design process.
1. Protein structure prediction using AlphaFold
In the 1990’s, a large number of developments were undertaken using combinatorial and high-throughput screening technologies, which accelerated drug discovery [13-15]. These technologies were widely adopted because they enabled the rapid synthesis and screening of large libraries, but unfortunately, no significant success was achieved and little progress toward the development of new molecular entities was made [16, 17]. 4For VS, consensus scoring can be used instead of a single scoring scheme to rank hit compounds to allow more diversity of the identified compounds (86). For example, in our SILCS-Pharm protocol, LGFE and RMSD are used together to rank compounds that pass our pharmacophore model filtering. Additional scoring metrics can include the DOCK or AUTODOCK scores (49, 50), or the average interaction energies from MD simulations, with many other variations available. The utility of the SSFEP approach is that the ΔΔG values for many modifications may be rapidly evaluated as the same trajectories from the original MD simulations of the hit compound are used in each case.
Aurigene Pharmaceutical Services Ltd. introduces Aurigene.AI™, an artificial intelligence (AI) and machine learning ... - Business Wire
Aurigene Pharmaceutical Services Ltd. introduces Aurigene.AI™, an artificial intelligence (AI) and machine learning ....
Posted: Wed, 03 Apr 2024 11:00:00 GMT [source]
To expand the range of structure-based docking applicability to those targets lacking high-resolution structures, it is also tempting to use AI-derived AlphaFold2 (refs. 99,100) or RosettaFold101 3D models, which already show utility in many applications, including protein–protein and protein–peptide docking102. Traditional homology models based on close protein similarity, especially when refined with known ligands103, have been used in small-molecule docking and virtual screening104, therefore AlphaFold2 is expected to further expand the scope of structural modelling and its accuracy. In a recent report, AlphaFold2 models, augmented by other AI approaches, helped to identify a cyclin-dependent kinase 20 (CDK20) small-molecule inhibitor, although at a modest affinity of 8.9 μM (ref. 105). More general benchmarking of the performance of AlphaFold2 models in virtual screening, however, gives mixed results. In a benchmark focused on targets with existing crystal structures, most AlphaFold2 models had to be cleaned from loops blocking the binding pocket and/or augmented with known ion or other cofactors to achieve reasonable enrichment of hits106. The recently developed AphaFill approach109 for ‘transplanting’ small-molecule cofactors and ligands form PDB structures to homologous AlphaFold2 models can potentially help to validate and optimize these models, although further assessment of their utility for docking and virtual screening is ongoing.
1. Structure-based Drug Design (SBDD)
Virtual screening (VS) is a computational technique used for screening large datasets of molecules, and has been successfully used to complement High Throughput Screening (HTS) for drug discovery [8, 20, 21]. The major aim of VS is to enable the rapid, cost-effective evaluation of huge virtual compound databases to screen for effective leads for synthesis and further study [22]. Virtual database screening can be applied to screen large libraries of compounds using various computational approaches to identify those entities likely to bind to a molecular target of interest [23, 24]. To a large extent, VS mitigates the problem of drug synthesis because it utilizes large libraries of pre-synthesized compounds.
The most promising approach in the present big data world is deep learning which was first used in the drug discovery process in 2012 QSAR machine learning challenge backed by Merck [110]. The results showed that deep learning models were true which can accurately predict the ADMET properties compared to traditional machine learning methods. Although, AI is an impressing method in identification of preclinical candidates in more cost and time-efficient manner, and the accurate prediction of binding affinity between a drug molecule and a receptor using AI remains challenging for quite a several reasons. Firstly, AI is a data mining method whose performance heavily relies on the amount and quality of the available data [4, 111]. Variability in the source of data especially those derived from different biological assays and lack of high-quality data from public databases presents difficulty in efficient AI learning [112, 113].
Biomacromolecular therapeutics, or so called biologics, need to be carefully formulated to maximize protein stability and minimize viscosity, so as to ensure both efficacy and safety for highly concentrated formulations (141). Toward maximizing stability, biologics can be formulated with excipients to help minimize aggregation and denaturation of the biologic in a solution formulation (142). To assist the rational selection of excipients for biologics, we developed the SILCS-Biologics protocol (143, 144) which combines SILCS-PPI and SILCS-Hotspots as described above to predict both PPIs that can contribute to protein aggregation and increased viscosity, and binding sites of excipients. This information is then combined to build a model for protein stability, aggregation and viscosity prediction. An overview of the V-SYNTHES algorithm allowing effective screening of more than 31 billion compounds in REAL Space or even larger chemical spaces, while performing enumeration and docking of only small fractions of molecules. Results of the many successful structure-based prospective screening campaigns have been published over the years covering all major classes of targets, most recently GPCRs, as reviewed in refs.
To overcome limitations and improve accuracy in terms of predicting potent leads, regular updates of tools and algorithms are needed. Database reliability and high quality validated experimental molecules is to be developed and updated because many pharmacophores do not pass biological activity process due to non-availability of good quality data sets. Databases should contain detail data on genomics and proteomics, high quality sequence information, physicochemical properties, and structures. In particular, like any computer assisted hypothetical system results must be validated in actual systems, and many lead molecules identified using CADD have failed to exhibit desired activities in biological systems [83, 84]. Several parameters must be met before potential compound to be approved as potent lead/drug, as it has to pass several pharmacological criteria.
The advent of fast and practical methods for screening gigascale chemical spaces for drug discovery stimulates further growth of these on-demand spaces, supporting better diversity and the overall quality of identified hits and leads. Real-world testing of MADE-enhanced REAL Space, and other commercial and proprietary chemical spaces will allow a broader assessment of their synthesizability and overall utility38,117,118. In parallel, specialized ultra-large libraries can be built for important scaffolds underrepresented in general purpose on-demand spaces, for example, screening of a virtual library of 75 million easily synthesizable tetrahydropyridines recently yielded potent agonists for the 5-HT2A receptor119. Despite amazing progress in basic life sciences and biotechnology, drug discovery and development (DDD) remain slow and expensive, taking on average approximately 15 years and approximately US$2 billion to make a small-molecule drug1. Although it is accepted that clinical studies are the priciest part of the development of each drug, most time-saving and cost-saving opportunities reside in the earlier discovery and preclinical stages. Preclinical efforts themselves account for more than 43% of expenses in pharma, in addition to major public funding1, driven by the high attrition rate at every step from target selection to hit identification and lead optimization to the selection of clinical candidates.
Computer-Aided Drug Design tools are now an indispensable part of drug discovery that have made key contributions to the development of drugs. In this editorial, I briefly provide an overview of CADD emphasizing its potential and invite authors from academia and the pharmaceutical and biotechnology sector to present their research in this collection. Although the synthetic success rate for some of the commercial on-demand chemical spaces (for example, Enamine REAL Space) have been thoroughly validated20,21,22,23,24,26,42, synthetic accessibilities and success rates of other chemical spaces remain unpublished38.
Comments
Post a Comment